Blogs
To know about all things Digitisation and Innovation read our blogs here.
Data Transformation
Comparing Different Data Transformation Tools and Techniques
SID Global Solutions
27 December 2022
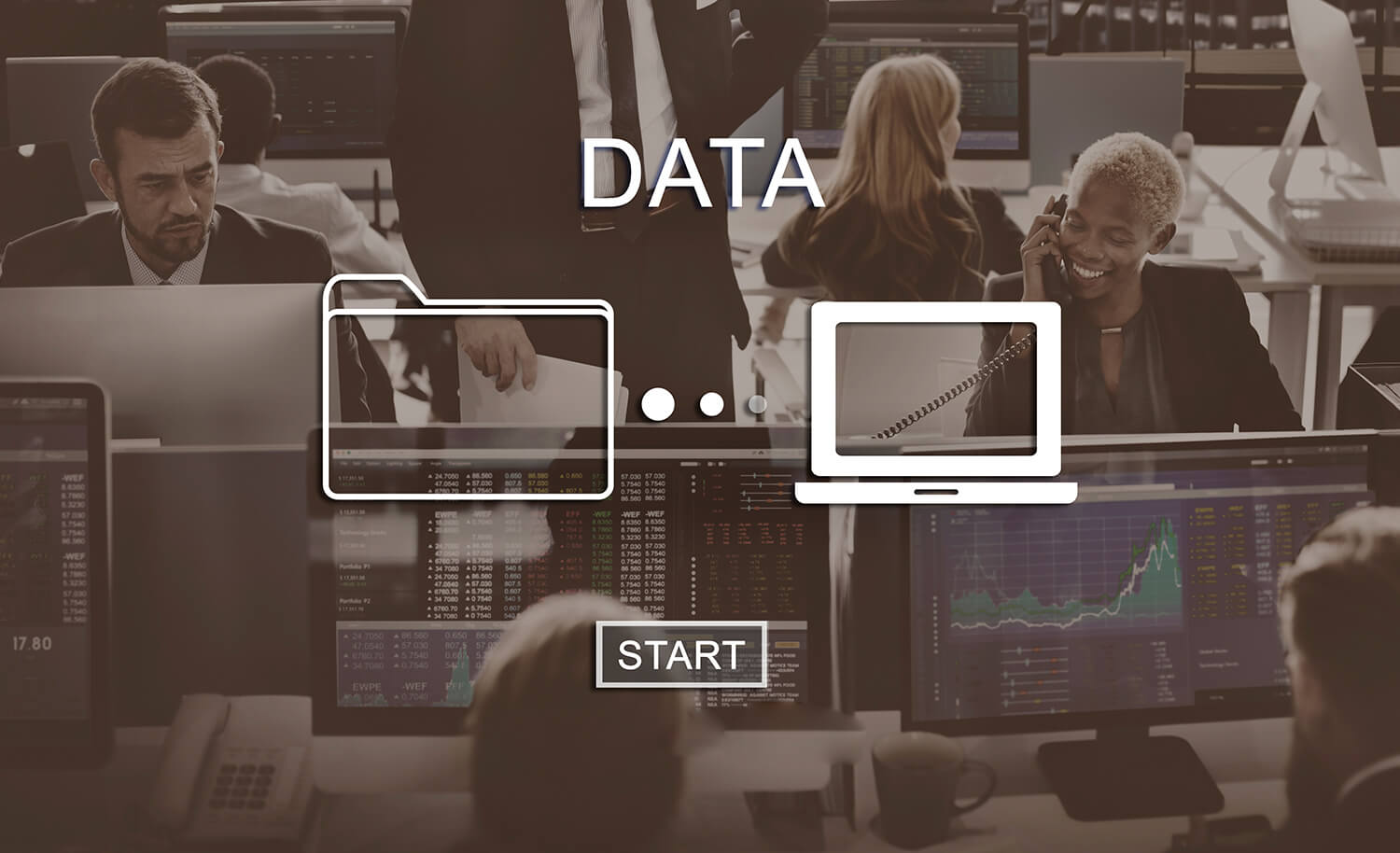
Overview
Data transformation is an essential part of any data analytics process, allowing businesses to turn raw data into useful insights. With the rise of Big Data and data-driven decision-making, having the right data transformation tools and techniques is essential to success.
In this blog post, we will compare different data transformation tools and techniques to help you determine which is best suited to your data needs.
Digital Transformation Tools and Techniques
We’ll consider some of the most popular approaches, such as ETL (extract, transform, load) and ETL tools, data integration, and data wrangling.
ETL (Extract, Transform, Load)
ETL, which stands for extract, transform, and load, is the most traditional data transformation tool. It is a process that extracts data from one source, transforms it into another format, and then loads it into the target system. The advantage of this approach is that it handles all the necessary steps from extracting data from its source to loading it into the target system.
ETL tools are used to automate the ETL process, simplifying the process and making it faster and more efficient. These tools typically include features such as data transformation, data cleansing, and data integration. They are designed to handle complex data transformation tasks, such as merging multiple datasets or dealing with complex data formats.
Data Integration
Data integration is the process of combining data from multiple sources into a single, integrated view. This approach to data transformation is often used by businesses that need to combine data from different sources in order to gain insights. For example, a company might need to combine customer data from multiple sources, such as an e-commerce platform and a CRM, in order to gain insights into customer behaviour.
Data integration tools are designed to automate the process of combining data from multiple sources. They typically include features such as data cleansing, data mapping, and data merging. They can also be used to transform data from one format to another, such as from a CSV file to an XML file.
Data Wrangling
Data wrangling is the process of manually transforming and cleaning data in order to make it easier to analyze. This process is often used to prepare data for machine learning and other advanced analytics techniques. It can involve tasks such as data cleansing, data transformation, and data enrichment.
Data wrangling tools are designed to make the process of data wrangling easier and faster. These tools typically include features such as data manipulation, data filtering, and data summarization. They can also be used to automate the cleaning and transformation of data, allowing data analysts to focus on the analysis of the data.
Conclusion
Data transformation is an essential part of any data analytics process. Different data transformation tools and techniques can be used to turn raw data into useful insights. ETL is the most traditional approach, while data integration and data wrangling can be used to combine data from multiple sources and prepare data for machine learning and other advanced analytics techniques. Choosing the right data transformation tools and techniques will depend on the needs of your business, so it is important to consider what is best suited to your data needs.